How Synthetic Data in Insurance Is Shaping Its Landscape
Naturally, gathered data poses security and privacy concerns, can be inconsistent, or lack diversity. However, data remains crucial for insurance providers in processes like risk assessment, claim management, and fraud detection. The challenges of using real-world data push insurers toward a safer solution—synthetic data. But how does it work, and where should you start?
Syntho offers a smart platform that leverages various synthetic data forms and generation methods, enabling organizations to turn data into a competitive advantage. This guide will show how synthetic data can address the major challenges insurers face, unlock significant benefits, and drive future innovation. At the end, we’ll provide a practical, step-by-step plan for integrating synthetic data into your operations. Stay tuned!
Table of Contents
What Is Synthetic Data and Its Role in Insurance?
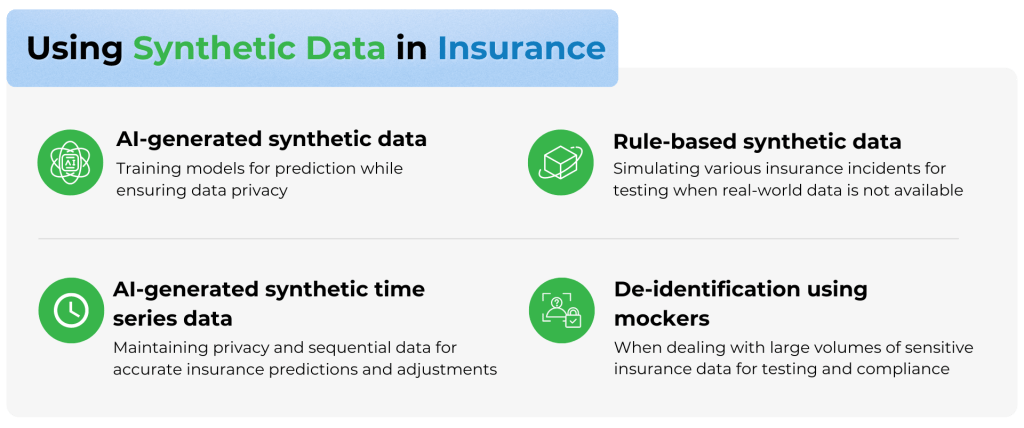
So, accurate risk assessment and informed decision-making require relevant data. While using information gathered from actual events and people might be insightful, it presents certain security and data privacy risks for insurance companies and their clients. That’s where synthetic data becomes a great alternative.
Synthetic data is artificially generated data that mimics the characteristics and patterns of real-world data. Insurance companies can use it to train AI models, validate ideas without exposing sensitive information, and for test data management.
In the realm of analytics, AI-generated synthetic data allows insurance companies to create datasets tailored to specific analytical needs. Insurers can use this data, for instance, to model customer behavior trends, conduct risk assessments, and even simulate rare events that may not frequently occur in real data, such as natural disasters or market crashes.
Artificially generated data is particularly beneficial for test data management. When insurance companies test their systems, they need a dataset covering a wide range of potential future cases. And since production data often lacks sufficient diversity or may not even exist, synthetic data helps ensure thorough testing coverage and identify potential issues before deployment.
De-identification is highly valued by insurance providers, and it is offered by platforms like Syntho. It involves removing all Personally Identifiable Information (PII) from datasets and replacing it with new, artificial identifiers (mockers). This allows insurance companies to use real data from their clients safely and ethically.
Depending on how it is generated, there are different types of synthetic data. Each type can help address specific challenges. Below, we present a table with the various synthetic data generation methods supported by the Syntho platform, along with examples of how they are used in the insurance industry.
Data generation method | Relevance | Example of use |
---|---|---|
AI-generated synthetic data | When accurate risk assessment and modeling are needed while ensuring data privacy. | Training a model to predict the likelihood of a claim based on customer profiles and past claims. |
AI-generated synthetic time series data | When sequential data is required for accurate predictions, and privacy must be preserved. | Analyzing patterns in annual claims to forecast future insurance needs or policy adjustments. |
De-identification using mockers | When dealing with large volumes of sensitive insurance data for testing and compliance. | Testing a claims processing system with fake but realistic data to ensure it handles different types of claims correctly. |
Rule-based synthetic data (using mockers and calculated columns) | When custom scenarios are needed or when real-world data is not yet available. | Simulating various insurance scenarios like high-risk accidents or complex claims to test policy underwriting rules. |
So, this is a brief overview of what synthetic data has to offer the insurance sector. Our following sections will provide a more detailed picture of how you can use synthetic data generation, starting with the most pressing challenges faced by insurers. Spoiler alert: it can help.
How Does Synthetic Data Solve the Biggest Data Challenges in the Insurance Industry?
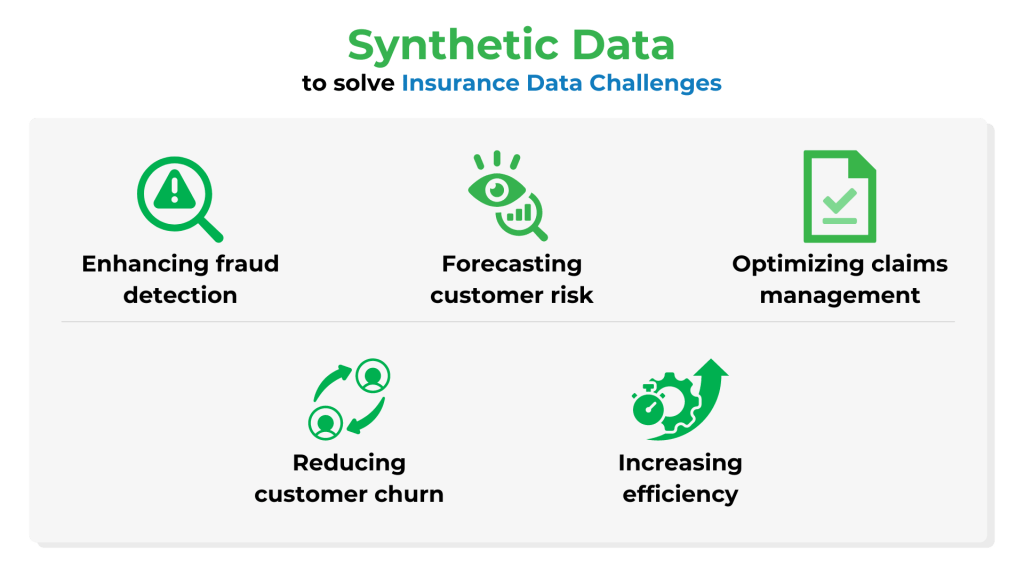
Insurance data challenges, unfortunately, are not limited to data privacy. As the world advances technologically, bringing both new opportunities and challenges, policyholders expect faster, more personalized service with increased security guarantees. To keep their customers satisfied and engaged, insurers need to adapt and evolve.
The challenges listed here are based on Syntho’s client experiences. If you don’t see the specific challenge you’re facing, feel free to reach out to us to discover how our platform can help.
Enhancing fraud detection
Forecasting customer risk
Customer risk assessment helps insurance companies tailor products, pricing, and coverage to better meet their clients’ needs. By analyzing historical data, insurers can forecast future outcomes such as the probability of health conditions, life expectancy, or behavioral risks that might influence the need for a policy.
Real-world datasets might not cover all possible scenarios, which can lead to incomplete or biased risk assessments. Additionally, using synthetic data allows insurance companies to overcome privacy constraints and provides a safer method to refine forecasting algorithms and improve risk management.
Optimized claims management and reducing customer churn
To reduce customer churn, insurance companies need to effectively manage claims and ensure customer satisfaction. Achieving this requires deep insights into consumer behaviors and preferences. However, accessing real-world data is often difficult, limiting the ability to capture all possible scenarios.
Synthetic data allows insurers to model and analyze complex customer behaviors without being limited. Thus, insurance companies can improve customer service, and discover new opportunities for personalized service to retain existing customers and minimize churn.
Increased efficiency through automated processes and analytics tools
Synthetic data is abundant, readily available, and can be used for training models, and making predictive analyses. This constant availability of data enables faster processing of claims, more thorough risk assessments, and quicker adjustments to pricing and marketing strategies. As a result, processes become more efficient, leading to lower operational costs.
To conclude, synthetic data helps insurance businesses grow faster, address security and privacy concerns, and satisfy clients along the way. Next, we will discuss specific ways synthetic data generation platforms can assist insurance providers.
Address your main insurance challenges with Syntho.
What Else Can Synthetic Data Generation Offer Insurance Providers?
Apart from addressing some of the biggest challenges faced by insurers, synthetic data also improves data accessibility, facilitates collaboration with external partners, aids in developing new systems, and simplifies data aggregation. How? Syntho has the answers.
Testing and developing new products
Ensuring an outstanding digital customer experience involves using customer data to test different scenarios. Insurers can either de-identify existing data to gain insights from real information or generate synthetic data to explore more diverse cases.
Syntho provides insurance companies with both synthetic data generation and de-identification features. We offer a PII scanner that can automatically identify and remove Personally Identifiable Information (PII) and Protected Health Information (PHI).
Insurance companies can also use rule-based synthetic data to simulate real-world scenarios during product testing and development, by applying predefined rules to generate accurate, diverse datasets without compromising sensitive data.
Additionally, synthetic data generation platforms like Syntho, which offer consistent mapping, ensure that synthetic data created from different datasets maintains consistent relationships across those datasets. It helps keep test data reliable in non-production environments by preventing inconsistencies. This ensures that the relationships between tables are accurate and useful for testing and software development.
All in all, it allows insurance companies to predict possible outcomes and mitigate risks before the launch.
Ensuring data privacy while maintaining accessibility
Insurance companies work with a lot of sensitive data. Due to privacy concerns and the high risk of real data being targeted for fraudulent activities, sharing information—even within the same organization—can be challenging. Synthetic data, on the other hand, can be easily exchanged between departments without any risk.
Collaborating with external partners
When insurance providers need to collaborate with an external company, such as a technology vendor, the challenge of data sharing often arises. Sharing personal customer information can be restricted by law but is necessary for developing or improving the systems and services insurers provide.
Synthetic data is a great solution here, since, as already has been established, it has no connection to real personal data and just mimics its characteristics.
A notable example of improved collaboration is our work with the Netherlands Chamber of Commerce (KVK) during their hackathon. By using synthetic data that replicated real commercial register information, the KVK ensured data privacy and compliance while fostering data-driven innovation. This approach enabled secure data sharing and unrestricted access for participants, allowing them to quickly develop and test solutions without having to handle sensitive information. The successful implementation underlines the value of synthetic data for collaboration with external partners and its effectiveness in secure data-sharing scenarios.
Data aggregation
Many insurance companies need to aggregate data from different sources, such as customer databases or claim tickets. By law, sensitive data cannot be stored as it is and must be anonymized to ensure compliance with privacy regulations.
To address this, companies can either anonymize the data directly or convert it into synthetic data, both of which preserve the utility of the information while protecting individual identities.
As you can see, synthetic data has a lot to offer, and platforms like Syntho provide insurers with various features to meet their needs. However, as technologies evolve, so do the tools. In the next section, we will briefly discuss the advances we can expect from synthetic data in insurance in the future.
Syntho can help you de-identify your datasets or generate new synthetic ones.
The Future of Synthetic Data in the Insurance Industry
How to Leverage Synthetic Data in Insurance Efficiently
While there is no single way to use synthetic data, certain considerations are essential when beginning data generation. This section draws on our expertise and Syntho’s client experiences, allowing us to highlight the key steps for efficiently starting to use synthetic data in the insurance industry.
- Identify data challenges holding back your business, such as privacy concerns or gaps in customer insights.
- Clearly establish what you want to achieve with synthetic data, whether it’s better decision-making, improved risk assessment, or enhanced product development.
- Choose a trusted synthetic data provider like Syntho that can deliver secure and reliable data generation.
- Ensure the tool provides the features you need, like a PII scanner or consistent mapping.
- When using the platform, ensure the synthetic data works seamlessly with your existing systems to keep operations running efficiently.
- Use the new data to achieve your goals.
- Regularly check the data to ensure it supports fair and unbiased business decisions.
- Continuously refine your synthetic data strategies to stay ahead of market changes.
- Partner with experts like Syntho to get the most out of synthetic data and navigate any challenges effectively.
And that’s how insurance providers can use synthetic data in the insurance industry efficiently.
Syntho Helps You Unlock Valuable Insurance Insights with Synthetic Data
Whether to use synthetic data is a choice, and all the insights above have shown that it’s the right one for the insurance industry. The next important step is to select the right provider. Syntho is a leader in artificially generated data, assessed by external experts like SAS for accuracy, privacy, and speed. We offer a variety of features, including advanced PII scanning to ensure sensitive information is identified and protected, consistent mapping for seamless data integration across different systems, and upsampling to create more balanced synthetic datasets that enhance the accuracy of predictive models.
As the industry continues to evolve, partnering with a trusted provider like Syntho ensures that you’re not just keeping up with the future of data but leading it. Book a demo today to learn how Syntho can transform your business and help you stay ahead in the competitive insurance market.
About the author
CEO & founder
Syntho, the scale-up that is disrupting the data industry with AI-generated synthetic data. Wim Kees has proven with Syntho that he can unlock privacy-sensitive data to make data smarter and faster available so that organizations can realize data-driven innovation. As a result, Wim Kees and Syntho won the prestigious Philips Innovation Award, won the SAS global hackathon in healthcare and life science, and is selected as leading generative AI Scale-Up by NVIDIA.
Explore Syntho's synthetic data generation platform
Fuel innovation, unlock analytical insights, and streamline software development — all while maintaining the highest data privacy and security standards.