Synthetic Data in Healthcare: Its Transformative Role, Benefits & Challenges
The lack of high-quality data and strict privacy regulations can hinder the use of AI analytics for disease identification, medical predictions, and clinical research. Synthetic data in healthcare offers an effective way to address these challenges at minimal cost.
Synthetic data enables healthcare innovation by letting organizations use an analog of real data without compromising privacy. Gartner predicts that by 2024, 60% of the data organizations use to train AI platforms will be synthetic, a significant increase from 1% in 2021.
Our team at Syntho will introduce you to the limitations and challenges of healthcare data usage. We’ll also discuss how to overcome these challenges with synthetic datasets.
Table of Contents
Key challenges to using real-world healthcare data
Healthcare organizations leverage data to make evidence-based decisions, enhance patient outcomes, and conduct medical research. However, companies often struggle with data scarcity and a lack of granularity, both of which hinder accurate predictions. This challenge is compounded by stringent security measures implemented to address privacy regulations.
Strict privacy and security regulations
Anonymization alone doesn’t ensure data privacy
However, traditional anonymized data often falls short in large datasets. Techniques such as data obfuscation and data masking techniques can erase most of the valuable information needed for data analysis. This challenges researchers who rely on detailed data for in-depth analysis and exploration.
Besides, the risk of re-identification still exists. Research shows that the de-identification of health records against up to 40 variables can be compromised when datasets include unique characteristics (like a rare disease or a specific medication).
Quality healthcare data is scarce
Healthcare organizations often lack data on patient symptoms, diagnoses, and treatment outcomes and face challenges with unobstructed data access. This deficiency limits the ability to capture clinical nuances essential for research.
Gartner predicts an increase in the use of synthetic data created with generative AI (in healthcare and other industries) to fill gaps in data availability. However, what data will be used to train generative AI models? That’s a valid question, as data scientists will require high-quality training data to achieve optimal results.
QA Datasets can be incompatible or low-quality
Health data can come from various sources in formats that may be incompatible with one another. Organizations have to combine structured Electronic Health Records (EHRs) with unstructured data from wearables, third-party software, and paper records.
Human errors and system glitches can affect data quality and impact the dependability of data analysis, impacting the data utility. This can lead to incorrect conclusions and misguided decisions.
Now that we’ve outlined the key challenges, let’s unpack how synthetic healthcare data can address them.
Gartner predicts an increase in the use of synthetic data created with generative AI (in healthcare and other industries) to fill gaps in data availability. However, what data will be used to train generative AI models? That’s a valid question, as data scientists will require high-quality training data to achieve optimal results.
How does synthetic data in healthcare help?
Synthetic data is artificially generated data points created with statistical models and algorithms.
The algorithms mimic all patterns and relationships of real-world data and create the synthetic.
This data generation model detects and learns about patterns in the real-world data and produces a synthetic data twin of the real datasets, preserving its statistical properties but replacing personally identifiable information (PII).
The role of artificial, AI-generated healthcare data can be transformative for healthcare innovation. Synthetic datasets offer an alternative when actual health data is unusable due to quality issues, inaccessible due to privacy constraints, and in cases where too little data exists for quality data analysis. Machine learning models trained on synthetic datasets aid the development of innovative solutions while safeguarding sensitive information. In fact, it offers multiple benefits for healthcare organizations and related businesses. Check the ROI of Syntethic data.
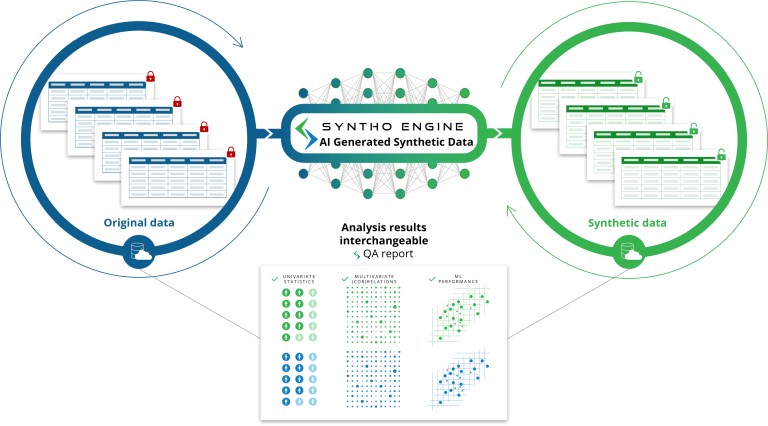
Benefits of synthetic data for healthcare organizations
Synthetic data has tremendous potential for healthcare providers, big pharma companies, and software developers. These advantages range from privacy and compliance benefits to cost reduction and streamlined research.
Synthetic patient data reduces privacy risks
Synthetic data allows healthcare organizations to share sensitive data without revealing PII. Consequently, it reduces the risk of disclosing sensitive information if there’s a data breach and, thus, limits the possibility of lawsuits and regulatory fines. Thanks to our focus on privacy in synthetic datasets, Syntho was recognized as one of the rising generative AI healthcare startups in 2023.
An example of maintaining privacy is how synthetic datasets handle patient visit dates. Visit dates are information that can be linked to a certain individual. To protect patient data and privacy, an ML model creates artificial visit dates but ensures they retain the pattern of the actual visits (e.g., the number of visits and the length of time between visits).
Synthesizing data saves time and resources
AI-generated synthetic data platforms eliminate the bureaucratic burden and expenses of accessing medical data. You’ll have fewer contractual terms to consider and governance processes to implement. This saves both time and reduces costs for healthcare providers and clinical research agencies. It also gives you a competitive advantage over companies that can’t access quality data as quickly.
Advanced platforms create data that protect you from compliance and privacy violations. They automatically assess privacy for critical metrics like the Identical Match Ratio (IMR) for exact matches, Distance to Closest Record (DCR) for similar matches, and Nearest Neighbour Distance Ratio (NNDR) for matching outliers. There are fewer compliance and privacy risks when working with data.
Syntho’s AI data generation solution won the 2023 Global SAS Hackathon in Healthcare and Life Sciences. Industry experts recognized our platform for its ability to provide hospitals with high-quality synthetic data for research, analysis, and innovation without compromising patient data and privacy. California’s leading hospital uses our artificial data generation platform to advance its research, including clinical trials.
Synthetic data can fill in data access gaps
Synthetic data can help when the real data is scarce and limited or there are issues with data access. Moreover, this data retains essential features and patterns of real data, preserving the original data’s statistical properties and proving invaluable for specialists in healthcare research data centers.
For instance, if a clinical trial managed by a US pharma company enrolls EU cancer patients, it might encounter legal obstacles when trying to obtain data from foreign healthcare organizations. Generative AI platforms can help get the necessary datasets without the red tape. Our partner, LifeLines, uses our AI data-generation solutions to provide synthetic data for healthcare research.
AI machine learning algorithms can train on artificial medical data. Our research verified that synthetic data can be used to train ML models cost-efficiently. Comparisons showcase comparable predictive capabilities to models trained on real-world data. Synthetic data also improves predictive accuracy by allowing data sharing. For example, models trained on data from two hospitals outperform those trained on data from only one hospital.
Synthetic data facilitates research on rare diseases
Synthetic data aids researchers in studying health and disease conditions in populations. Diverse data sampling expands testing opportunities in scenarios where obtaining large volumes of real patient data is challenging or impossible.
Erasmus MC, University Medical Center, leverages our synthetic data generation platform to use synthetic patient EMR data for advanced analytics. They emphasize that our datasets mirror the statistical properties of real data, all without disclosing any personally identifiable information.
None of this means artificial data is always safe to use, and not all synthetic data appears to be valuable. You may run into technical limitations, such as challenges in synthesizing hierarchical data, data biases, and balance problems. On top of that, stakeholders must meticulously examine synthetic data’s validity to prioritize what is essential for each specific use case and effectively manage expectations when they generate synthetic data.
Luckily, we know how to deal with these challenges. Syntho’s synthetic data engine works with all structured data types and is easily deployable to on-premise infrastructures and private clouds. We help generate data for use cases in healthcare and other businesses.
For example, we used the SAS Viya analytic platform for synthetic data validation to establish that synthesized health data mirrors real-data quality in terms of correlations, model performance, and variable importance. The Area Under Curve (AUC) score boosts predictive accuracy from 0.74 to 0.78 when synthesizing data from multiple hospitals (compared to the initial system’s results).
Syntho synthetic data innovations for healthcare analytics
Generating synthetic data is a game-changer for healthcare analytics systems. It bridges data access gaps, improves disease detection algorithms, and enables data-driven medical research. Furthermore, a synthetic data approach significantly mitigates compliance and privacy challenges.
Healthcare data is more complex and time-sensitive than data in most industries. That’s why organizations should work with a reputable and trustworthy healthcare data platform provider. The possibilities are nearly boundless when you have a reliable technical partner. Syntho, with its Syntho Engine, stands at the forefront of the AI-generated synthetic data field. We’re focused on addressing current technological challenges and exploring new, groundbreaking applications in healthcare data analytics.
Want to learn more? For more information, download and explore our HealthCare report or schedule an intro call.
About Syntho
Syntho provides a smart synthetic data generation platform, leveraging multiple synthetic data forms and generation methods, empowering organizations to intelligently transform data into a competitive edge. Our AI-generated synthetic data mimics statistical patterns of original data, ensuring accuracy, privacy, and speed, as assessed by external experts like SAS. With smart de-identification features and consistent mapping, sensitive information is protected while preserving referential integrity. Our platform enables the creation, management, and control of test data for non-production environments, utilizing rule-based synthetic data generation methods for targeted scenarios. Additionally, users can generate synthetic data programmatically and obtain realistic test data to develop comprehensive testing and development scenarios with ease.
About the author
CEO & founder
Syntho, the scale-up that is disrupting the data industry with AI-generated synthetic data. Wim Kees has proven with Syntho that he can unlock privacy-sensitive data to make data smarter and faster available so that organizations can realize data-driven innovation. As a result, Wim Kees and Syntho won the prestigious Philips Innovation Award, won the SAS global hackathon in healthcare and life science, and is selected as leading generative AI Scale-Up by NVIDIA.
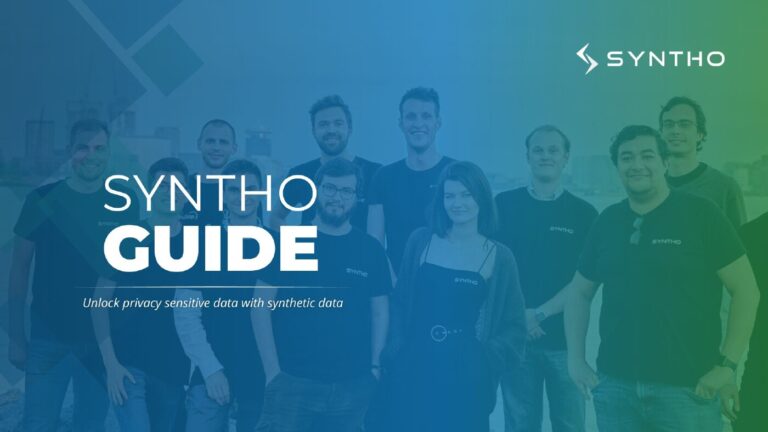
Save your synthetic data guide now!
- What is synthetic data?
- Why do organizations use it?
- Value adding synthetic data client cases
- How to start