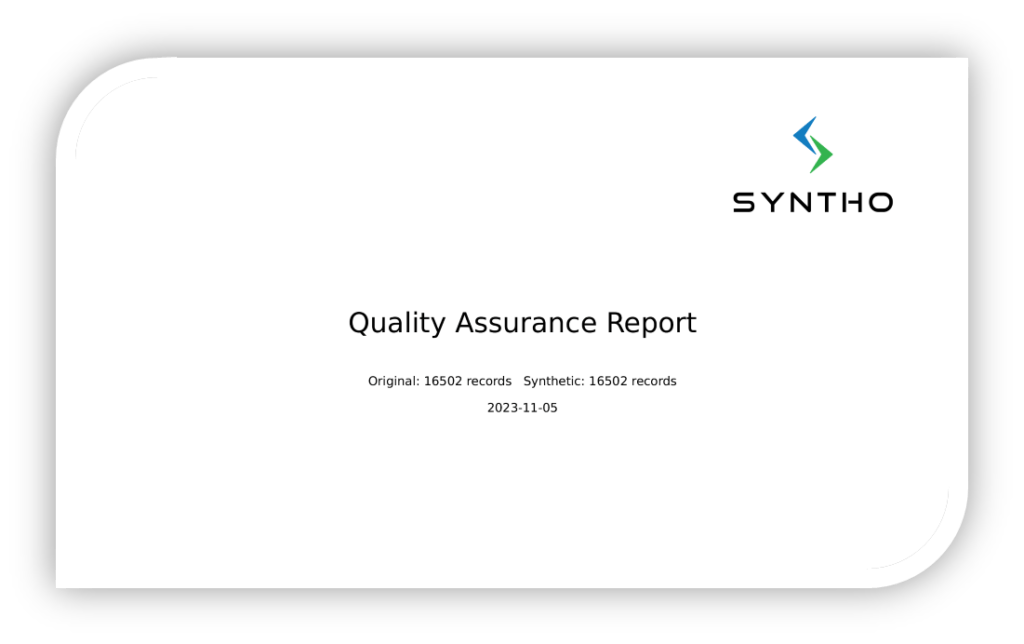
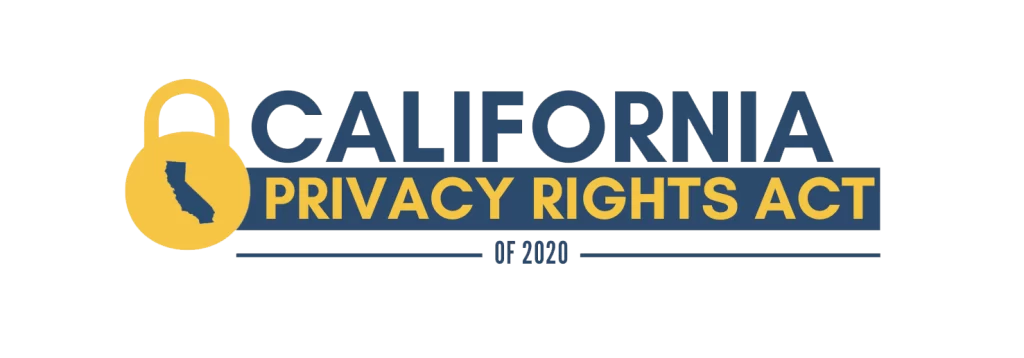
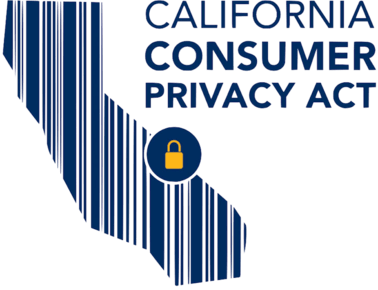
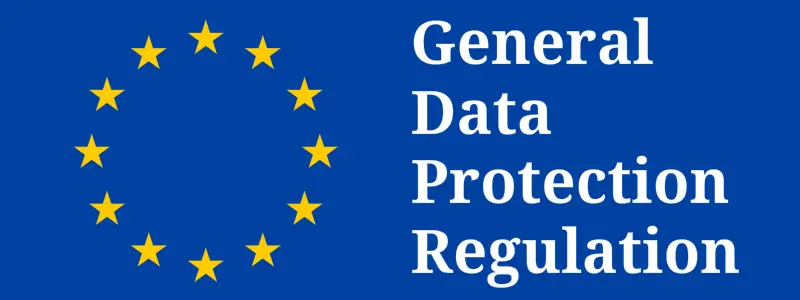
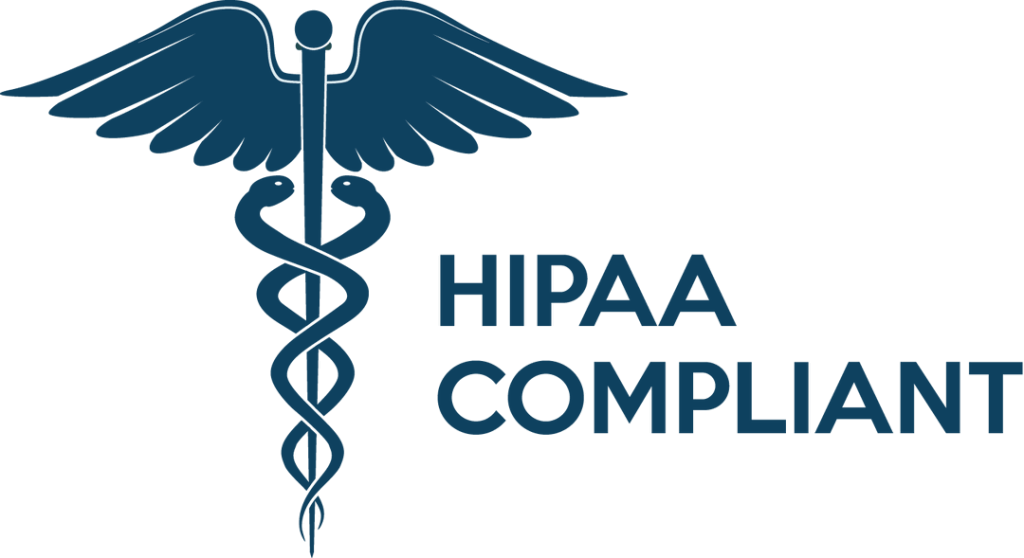
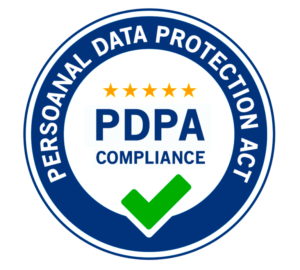
Why do organizations need QA reports?
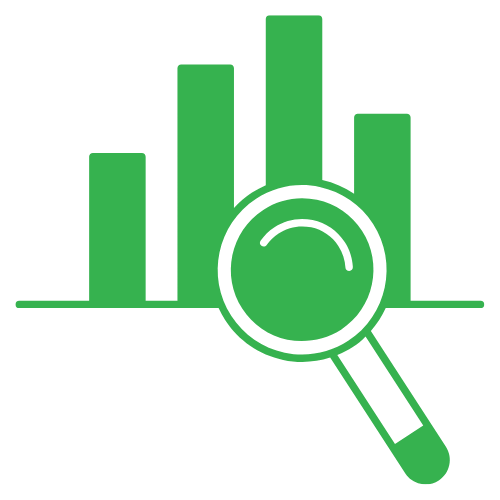
Industry-standard benchmark
Reliable and accurate synthetic data is a critical feature for synthetic data solutions. Our platform is aligned with industry standards, which provide robust benchmarks, models, and metrics.
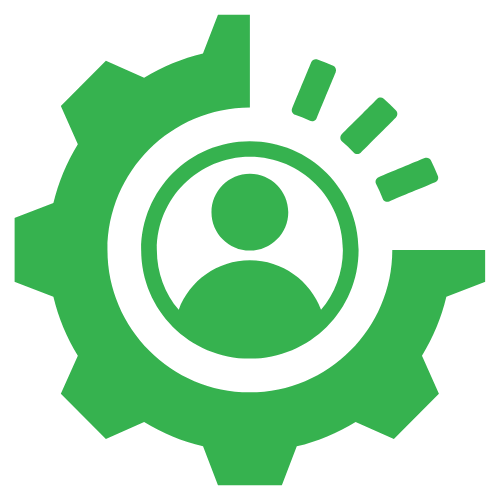
Assess synthetic data utility
Evaluating the quality of synthetic data involves measuring how accurately the generated data retains the statistical properties of the original dataset. This assessment ensures that the synthetic data reflects the same patterns, distributions, and correlations as the real data.
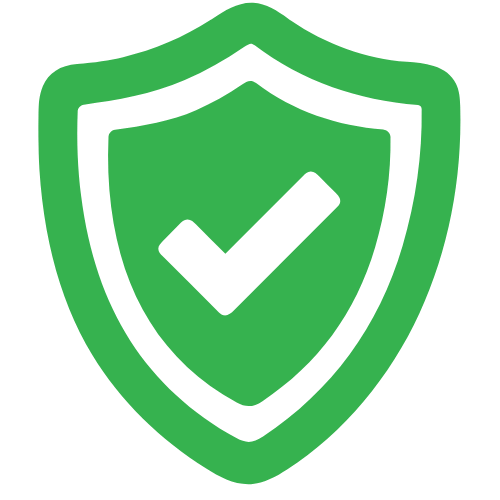
Privacy protection matrix
Privacy protection metrics measure the protection of the generated synthetic data in terms of privacy, offering a clear assessment of how well sensitive information is protected in the generated data.
Introduction to quality assurance report
Synthetic data utility metrics
Synthetic data privacy metrics
1. Identical Match Ratio (IMR)
“Exact matches”
Demonstration that the ratio of the synthetic data records that match a real record from the original data is not significantly greater than the ratio that can be expected when analyzing the train data.
Property: Considers identical records
2. Distance to Closest Record (DCR)
“Similar matches”
Demonstration that the normalized distance for synthetic data records to their nearest actual record within the original data is not significantly closer than the distance that can be expected when analyzing the train data.
Property: Considers “similar” records
3. Nearest Neighbour Distance Ratio (NNDR)
“Matching outliers”
Demonstration that the distance ratio between the nearest and second-nearest synthetic record to their closest record within the original data is not significantly closer than the ratio that is to be expected for the train data.
Property: Considers outliers
- Compare the accuracy of our synthetic data with real-world datasets
- Side-to-side comparison of our synthetic data mirroring patterns and characteristics
Real data and synthetic data comparison
Explore what synthetic data looks like and review a sample QA report
Report generation in 2 steps
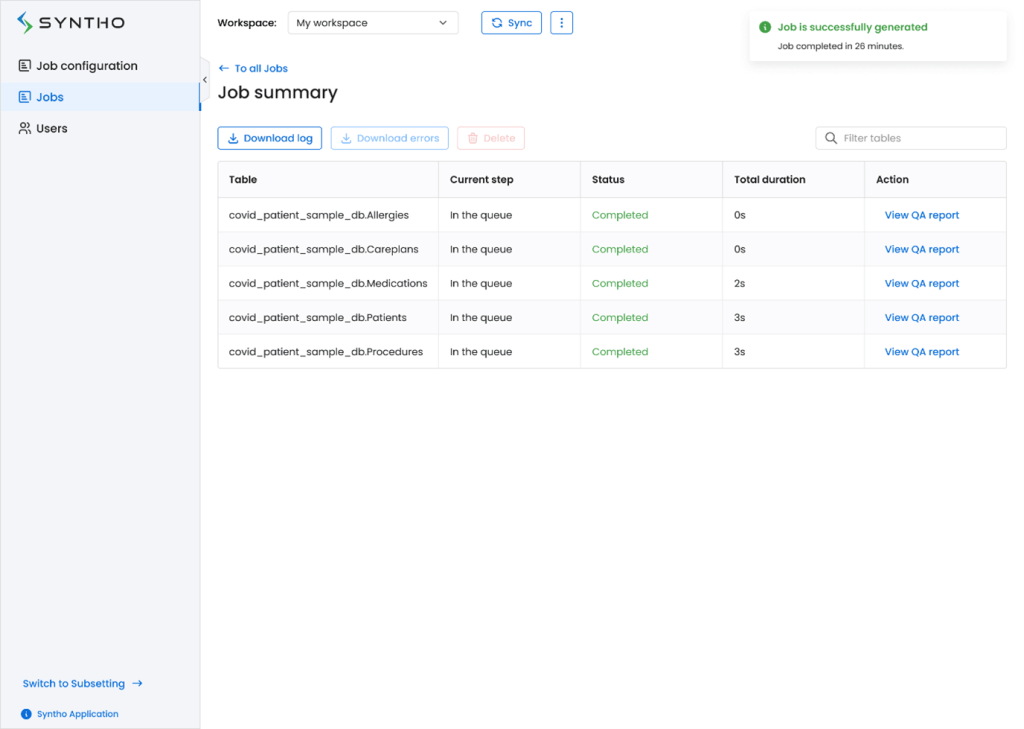
- A QA report can be automatically generated
- You can download the report in PDF format
Other features from Syntho
Explore other features that we provide
Frequently asked questions
What is data utility?
Data utility refers to how well a dataset meets the needs of its intended use. It encompasses accuracy, completeness, consistency, reliability, and relevance. High-quality data is accurate and free from errors, inconsistencies, or duplications, ensuring it can be effectively used for analysis, decision-making, and operational purposes.
What is synthetic data utility?
Synthetic data quality pertains to how closely synthetic datasets mimic real-world data’s statistical properties and characteristics. It evaluates the fidelity of the generated data, including its accuracy, reliability, and relevance, ensuring that synthetic data is a valid substitute for actual data in various applications.
What is a quality assurance report?
It is a synthetic data quality evaluation displayed in quality assurance and demonstrates the accuracy, privacy, and speed of the synthetic data compared to the original data. It provides a detailed analysis of the synthetic dataset, including metrics for accuracy, privacy, and performance, ensuring the data meets high standards.
Why do we provide a quality assurance report for every generated synthetic data set?
At Syntho, we understand the importance of reliable and accurate synthetic data. That’s why we provide a comprehensive quality assurance report for every synthetic data run. Our quality report includes various metrics such as distributions, correlations, multivariate distributions, privacy metrics, and more. This way, you can easily assess that the synthetic data we provide is of the highest quality and can be used with the same level of accuracy and reliability as your original data.
What do we assess in our quality assurance report?
Our quality assurance report evaluates:
- Accuracy: How closely the synthetic data matches the statistical properties of the original data.
- Privacy: Measures taken to ensure sensitive information is protected and not disclosed.
- Speed: The efficiency of the synthetic data generation process and its performance in real-time applications.
Why are synthetic data privacy metrics relevant?
Synthetic data privacy metrics are crucial because they asses if generated data does not reveal sensitive or personally identifiable information.
Challenges of synthetic data generation
- Maintaining Data Fidelity: Ensuring that synthetic datasets accurately reflect the statistical properties of real-world data.
- Balancing Privacy and Utility: Generating data that is both useful for analysis and secure from privacy risks.
- Handling Complex Data Relationships: Accurately modeling intricate relationships and dependencies in the data.
- Performance and Scalability: Efficiently generating large volumes of high-quality data in a timely manner.
Benefits of high-quality synthetic data
High-quality synthetic data offers several benefits:
- Enhanced Privacy: Protects sensitive information while providing valuable insights.
- Improved Accuracy: Provides a reliable alternative to real data for testing and training data for machine learning models.
- Cost Efficiency: Reduces the need for extensive data collection and management.
- Increased Flexibility: Allows for the creation of diverse datasets tailored to specific requirements or scenarios.
How do we measure the quality of synthetic data?
- Statistical Comparisons: Evaluating how well the synthetic data replicates the statistical properties of the original data.
- Privacy Metrics: Assessing the effectiveness of privacy protection measures.
- Utility Testing: Determining how well the synthetic data performs in real-world applications, such as training data for machine learning models.
Strategies for ensuring the quality of synthetic data
- Quality Assessment: Regularly evaluate synthetic datasets using statistical properties and privacy metrics to ensure accuracy and reliability.
- Robust Generation Techniques: Employ advanced algorithms and methods in the synthetic data generation process to maintain fidelity and relevance.
- Continuous Improvement: Regularly update and refine synthetic data generation techniques to address emerging challenges and enhance the quality of the synthetic data.
- Validation with Existing Data: Compare synthetic data against actual data to verify its accuracy and usefulness in practical scenarios.
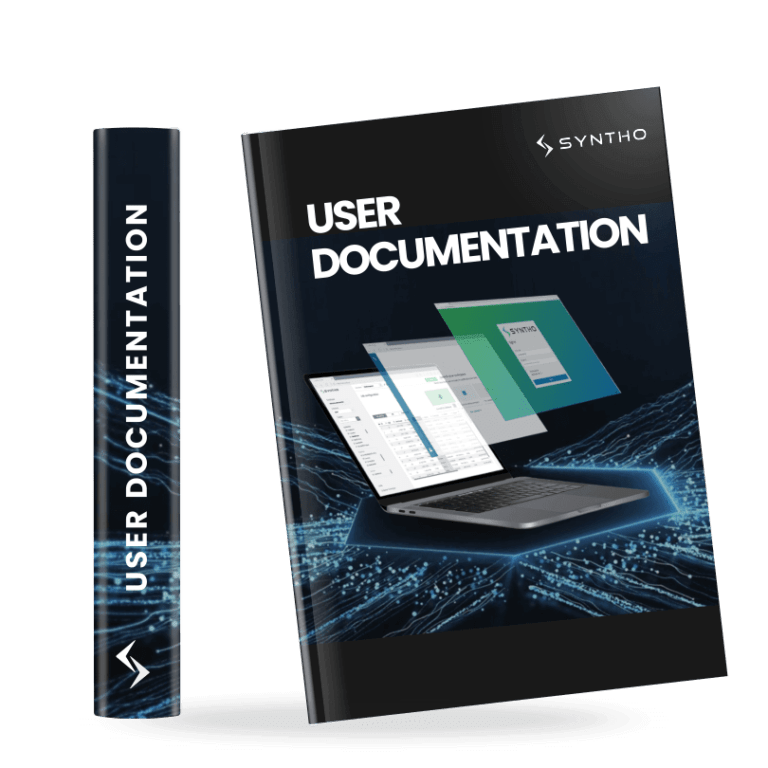
Access Syntho’s User Documentation!
- Getting started
- Deployment and connectors
- User interface
- Features
- User roles and support