Key benefits of using mock data
Substitute real values with mock values to de-identify data and enhance privacy
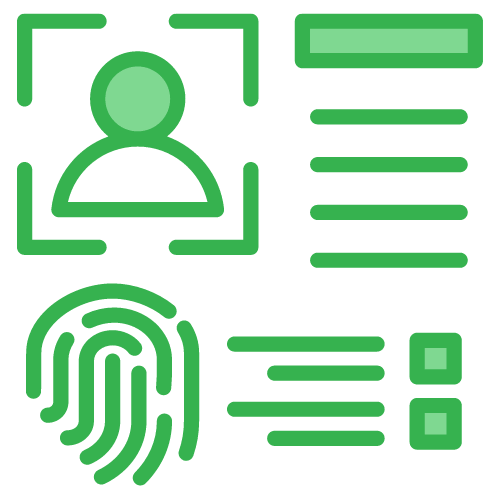
No PII and PHI data in the dataset
Organizations need easy-to-use and practical tools to exclude PII or PHI from their datasets to protect individual privacy and comply with data protection regulations. It helps to mitigate the risk of data breaches and ensure compliance with legal standards.
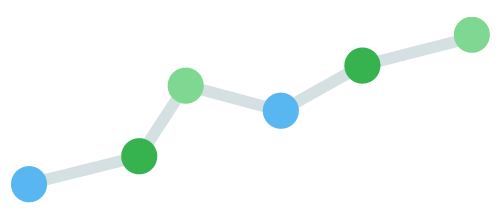
Representative data replacement
Mock data accurately reflects the characteristics of real data. It allows organizations to replace data with representative alternatives in minutes.
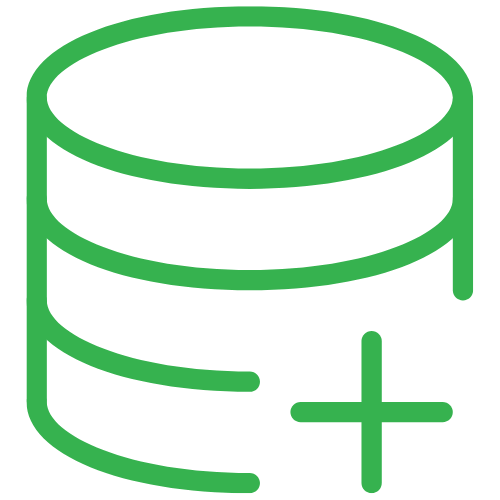
Complex data creation from scratch
Advanced data creation with rule-based synthetic data accurately simulates real-world scenarios. Enable the creation of highly realistic datasets from scratch, incorporating complex logic, relationships, and dependencies.
Check our User Documentation here
Why Syntho mockers are more advanced
Provides a wide variety of mockers capable of producing synthetic data
Syntho supports 150+ different mockers
Syntho supports default mockers such as first name, last name, social security, and phone number mockers, ensuring comprehensive and customizable data creation for diverse needs.
Advanced mockers
Advanced mockers are configurable mockers that enable users to fine-tune data according to their specific needs. Examples include the custom text mocker, which generates customizable strings containing letters, numbers, and symbols, and the Uniform Distribution Mocker, which allows users to set minimum, maximum, and precision to generate numerical values that follow a specific distribution.
Rule-based mock data
Rule-based mock data allows users to generating data based on predefined rules & logic or based on other columns in your database. Various formulas can be used to perform a wide range of operations on data, from simple arithmetic to complex logical and statistical computations. This ensures that the data adheres to specific patterns and constraints and allows for the creation of highly accurate and contextually relevant data.
Multilanguage support
Syntho supports each mocker in over 80 languages and different alphabets. We are supporting a diverse range of linguistic and regional needs.
How to apply mockers
Create synthetic data that enhances the volume and diversity of your data
Synthetic mock data in 3 steps
1. Identify PII
Scan PII automatically with our PII Scanner via the “PII” tab or identify columns that you would like to mock via the “Job Configuration” tab.
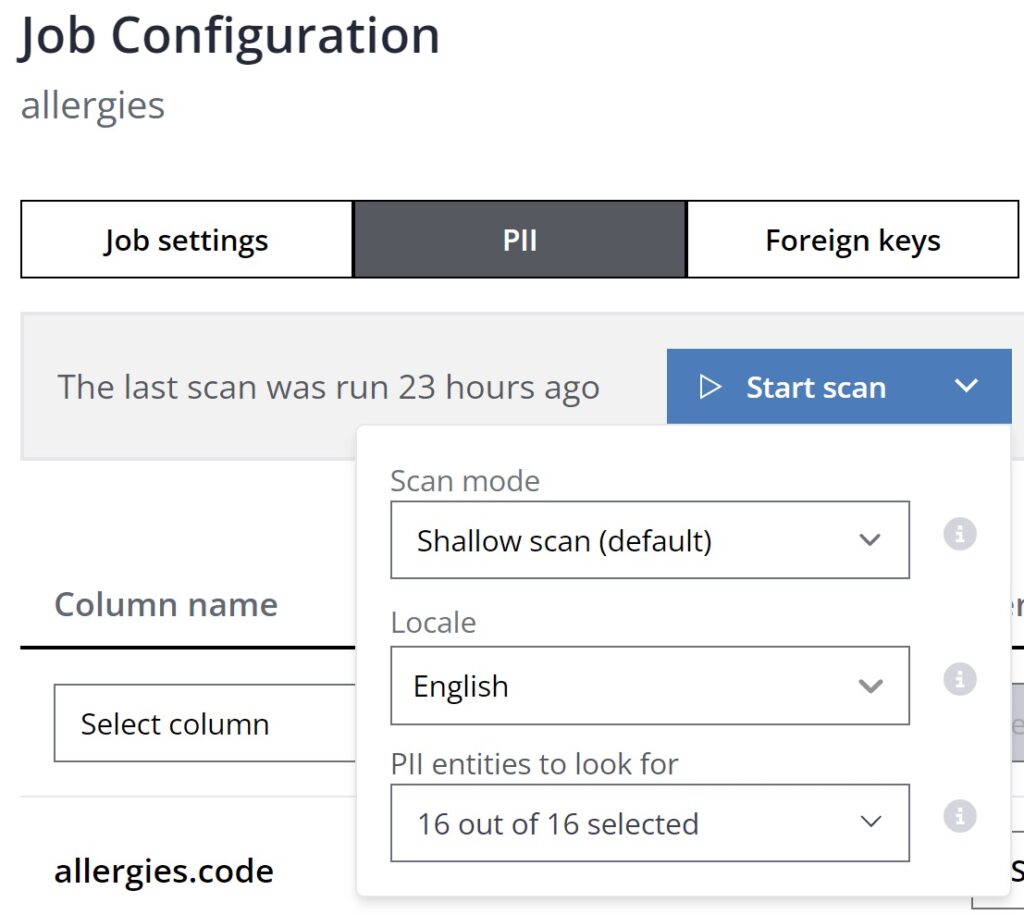
2. Select Mockers
Confirm the mocker by our PII scanner suggested mocker automatically or configure mockers on column level.
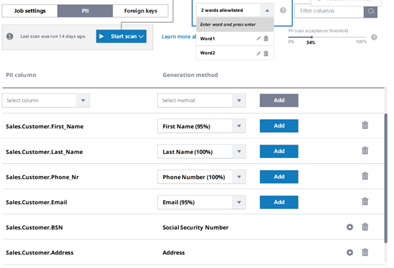
3. Confirm Mocker
Confirm the selected mocker to a column via the PII or Job Configuration tab. This allows users the flexibility to spot columns and apply mockers accordingly.
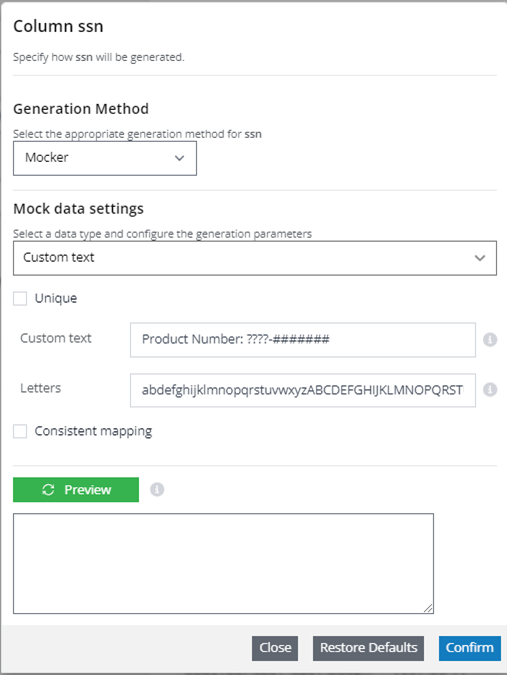
Other features from Syntho
Explore other features that we provide
Frequently asked questions
Substitute sensitive PII, PHI, and other identifiers with representative Synthetic Mock Data that follow business logic and patterns.
PII stands for Personal Identifiable Information. PHI stands for Personal Health Information and is an extended version of PII dedicated to health information. Both PII and PHI are identifiers and relate to any information that can be used to distinguish or trace an individual’s identity directly. Here, with identifiers, only one person shares this trait.
- First name
- Last name
- Phone number
- Social Security Number, SSN
- Bank number, etc.
PII, PHI, and other direct identifiers are sensitive and can be spotted manually or automatically with our PII scanner to save time and minimize manual work. Then, one can apply Mockers to substitute real values with mock values to de-identify data and enhance privacy.
Build better and faster with synthetic data
Unlock data access, accelerate development, and enhance data privacy. Book a session with our experts now.