Unlocking the Power of Synthetic Data in Healthcare: Interview with Experts
What drives healthcare forward, putting patients first and igniting scientific breakthroughs while keeping costs in check? It’s a wealth of data. With AI and advanced analytics, we’re reshaping healthcare through data, from research to market strategies to patient care.
Yet, in this data-driven era, challenges stand out. Quality issues, data scarcity, and legal obstacles create a complex landscape. Fortunately, there are cures. Leveraging synthetic data in healthcare stands as a viable solution.
I’m Uliana Krainska, a Synthetic Data Consultant at Syntho. I invite you to join me for an engaging discussion with industry leaders:
- Wim Kees Janssen, CEO & Co-founder, Syntho
- Frederick Droppert, Legal Professional, BG.legal
- Edwin van Unen, Principal Analytics Consultant, SAS
This interview delves into insights, success stories, and practical solutions, highlighting the potential of synthetic data in healthcare. So, let’s dive into our first question.
What are the main data-related challenges in healthcare today?
Wim: Well, in the healthtech sector, there’s a strong push to bring value to patients, to really make a difference in treatments, and to stand out from the competition through innovation. The main challenge we’re facing right now is using data-driven innovation to alleviate pressure on healthcare staff and create value.
Accessing the data we need is a huge obstacle, especially in healthcare, where data is sensitive. Without access to that data, building solutions become a non-starter, holding back progress and stopping us from delivering value. So, our primary focus is figuring out how to overcome these barriers and get the data we need to develop data-driven healthcare solutions.
Do you agree that in healthcare, it’s advanced analytics and machine learning (ML) techniques that are mostly driving innovation now? If so, how can companies use them effectively to beat the competition and bring value to all healthcare stakeholders?
Edwin: That’s true. But analytics and ML aren’t new concepts. While we talk about cutting-edge technologies like synthetic data and generative AI, the reality is that these methods have been around for quite some time—decades, even. What I often see, though, is that many organizations struggle to translate analytics and modern machine-learning models into tangible outcomes. The problem lies in the lack of an end-to-end process, from data to decision-making. This is where the analytics lifecycle comes into play.
Can you please talk more about this analytics lifecycle in healthcare? What does this process entail?
Edwin: Sure. The analytics lifecycle consists of three crucial steps.
- Data. It’s first and foremost. Accessing quality data and improving its quality further is critical.
- Model building. It involves running numerous experiments to determine the best approach and building multiple models quickly before deciding which ones to put in production.
- Deployment. Models must be deployed in a production setting to realize their full value, whether in real-time, batch, or streaming processes.
There should be smooth transitions between these steps to create an infinite loop from data to modeling to deployment and back again. Governance is also crucial at every phase as it ensures proper oversight of both data and models.
The problem here is that, unfortunately, many models fail to make it to the bedside, as I call it. If organizations don’t have this framework in place, innovation can’t reach the patient and bring that exceptional value we’re talking about. Lack of the end-to-end process, when something is missing at any of the steps, is the root of the problem. That’s where synthetic data generation and robust AI systems can shake things up.
What about another challenge you mentioned—accessing data in healthcare? What are the legal complexities surrounding data access and sharing?
Frederick: Data privacy laws like HIPAA in the US and GDPR in the EU loom over your every move. These acts are important as they set strict rules for protecting and using personally identifiable information (PII) and protected health information (PHI). However, they often feel like hurdles when you’re trying to access and share data for healthcare purposes.
Cross-border data transfer adds another layer of complexity. Healthcare organizations often lack vital data on patient symptoms, diagnoses, and treatment outcomes. That’s why they gather data from different countries for research and analysis. But it’s not as simple as it sounds. You’ve got to deal with contractual obligations, juggle consent requirements, and ensure anonymization standards are up to par.
The concept of data ownership also contributes to the complexity. While outright ownership may not always be clear, asserting control over data usage and sharing is essential.
The legal landscape concerning healthcare data access and sharing is full of twists and turns. Navigating it requires a keen eye for detail, a deep understanding of compliance, and a whole lot of patience.
Luckily, synthetic data has emerged as a potential solution. Could you please share how synthetic data can tackle the challenges you mentioned?
Wim: Synthetic data is truly a game-changer in addressing these challenges. It brings value to production, benefiting patients, customers, and stakeholders alike—all while maintaining strict privacy standards.
At Syntho, we offer a comprehensive solution that brings together various synthetic data generation methods under one roof.
This approach allows organizations to explore multiple solutions depending on their specific use cases. Whether it’s AI-generated data, de-identification techniques, or mock data, Syntho offers flexibility and versatility. We work closely with our clients to identify the best approach for their needs, ensuring the seamless and efficient transition from data to value.
You’ve talked about specific use cases. How exactly is synthetic data applied in healthcare now?
Edwin: That’s a great question. Synthetic data has been used in hundreds of cases spanning multiple industries, not just healthcare. Let me give you some specific scenarios where synthetic data shines.
First, synthetic data can fill the gaps when real data is scarce or nonexistent. This is particularly useful when creating test data or building demos, as organizations can showcase their solutions without relying on actual data.
Second, synthetic data comes in handy when augmenting existing datasets. Take fraud detection, for example. By generating synthetic cases of fraudulent activities, organizations can enhance their datasets and train robust models to identify fraudulent behavior early on.
With synthetic data, organizations can also create specialized datasets for testing purposes. Whether designing specific scenarios or generating unique cases, synthetic data provides the flexibility to test apps and systems thoroughly.
Lastly, synthesizing complete datasets or databases is one of the most compelling use cases of synthetic data. It’s when datasets are created from scratch to mimic the characteristics of real data while preserving privacy. This approach helps you build models faster and consolidate data from multiple sources. By synthesizing data from many hospitals or institutions, organizations can create comprehensive datasets for analysis and model training, overcoming legal barriers associated with data sharing.
That sounds promising. However, are there any obstacles that could hinder the widespread use of synthetic data in different scenarios? Legal regulations are the first concern that comes to mind…
Frederick: Once you’re dealing with data that needs to be de-identified, suddenly, you’re back in the world of GDPR compliance. Thankfully, in Europe, particularly in the Netherlands, regulatory bodies recognize synthetic data as a viable means of de-identification.
Once you’ve got synthetic data, it’s not personal data anymore, which means it’s not under the control or ownership of any specific company. This opens up some exciting possibilities. You can collaborate with other companies, combining datasets to uncover even more insights about patients.
Of course, the GDPR compliance requirement is still there. But in Europe, we’ve got some options to work with. It’s a bit more complex in the US, where state laws vary. Overall, there’s still a lot of potential here. Synthetic data offers a new pathway to explore and innovate while complying with the regulations.
Wim: I’d like to build on what Frederick just said and mention the Syntho platform’s approach as an example. I’m talking in particular about our intelligent data de-identification strategy. The smart de-identification process anonymizes data by using AI-generated synthetic mock data. This enables organizations to convert sensitive information into compliant, non-identifiable data through the following steps:
- Our de-identification software analyzes existing datasets, identifying personally identifiable information (PII) and protected health information (PHI).
- Organizations can selectively replace sensitive data with artificial information as needed.
- The tool generates new datasets containing compliant data.
This technology facilitates secure collaboration and data exchange among organizations. And it’s particularly helpful in ensuring data compliance across multiple relational databases.
What’s more, the smart de-identification process we designed preserves data relationships through consistent mapping. Companies can use the generated data for in-depth business analytics, training ML models, and testing.
What legal advice would you give to organizations that think about implementing synthetic data solutions in their healthcare initiatives?
Frederick: Before implementing any synthetic data solutions in healthcare, it’s crucial to pause and think it through. Start by asking yourself why you want to use synthetic data and how it can resolve your organization’s challenges. Once you’re on board with the idea, consider whether you’re dealing with digital assets or exploring other applications of synthetic data. Who owns the data you’ll be using? These are essential questions to ask as you embark on this journey.
Edwin’s suggestion about governance strategies throughout the lifecycle process is spot-on. Running risk assessments early on, even at the brainstorming stage, is key. Their results will guide you as you tackle regulatory compliance in your specific context.
The EU has recently adopted AI regulations, which makes liability critical, especially as you transition from synthetic to real data for model training. The EU AI Act categorizes AI-related technology by risk levels, ranging from “unacceptable” to low hazard. You must understand liability implications down to the last detail.
Security remains paramount as well, even with synthetic data, as it can be misused if it ever gets into the wrong hands. So, data must be kept as safe as ever.
Finally, pay close attention to contractual obligations regarding data usage and confidentiality. Sometimes, they extend beyond the data itself to include its inherent value. Seek guidance from legal, cybersecurity, and AI experts to stay on top of all these considerations.
Wim, can you share any specific challenges, aside from legislative ones, that your clients anticipate when introducing synthetic data into their workflows? How can you help mitigate them?
Wim: One key concern is the accuracy of synthetic data compared to real-world datasets, especially with AI-generated synthetic data. To tackle this, we conduct rigorous analyses to validate synthetic data’s accuracy and reliability against authentic datasets.
Moreover, explainable AI is pivotal in our implementation strategy. By sticking to the principles of explainable AI, we make AI models more transparent so stakeholders can understand the rationale behind AI-driven decisions. This builds trust and confidence that synthetic data is reliable and useful.
Complex data types, such as time series data, create another hurdle. Organizations may face difficulties understanding and generating synthetic data, particularly with complex data types like time series data and scaling synthetic data generation to production levels. We’re ready to guide our clients through any challenges to facilitate the integration of synthetic data into their workflows. Furthermore, Syntho’s platform is user-friendly, so our clients don’t actually grapple with such issues.
Let’s wrap it up by discussing some of the best practices for implementing synthetic data strategies in healthcare. Wim, I’m sure you’ll be glad to share some.
Wim: Sure. First, you have to figure out what you need the synthetic data for. If, for instance, it’s just for testing or demos, you might not need it to be super accurate. However, accuracy should be your top priority if you’re using it to train AI models.
We’ve worked with partners like Intel to test how well models built on synthetic data perform compared to those built on real data. The results were really promising. They only confirmed that synthetic data is effective in model training.
When it comes to using synthetic data, it’s best to integrate it early on, right after you’ve gotten access to the original data. By starting with synthetic data, you can get a head start on model development and innovation without waiting months to access real data. This makes the process smoother. You can then build and test your models using synthetic data and pick the best ones for real-world use.
But it’s not just about building models; you also need to make sure everything is done properly and securely. That means having rules and checks in place at every step, from data access to model deployment.
Overall, synthetic data offers a game-changing advantage for healthcare organizations, pharmaceutical companies, and software developers. Creating statistically accurate data that shields sensitive patient information slashes the risk of privacy breaches and hefty fines.
This innovation doesn’t just save time and resources by sidestepping bureaucratic hurdles; it also opens doors for research where access to authentic data is limited. It empowers the study of rare diseases and enhances predictive accuracy in medical research.
While challenges like data biases and legislative cautiousness do exist, solutions like Syntho’s synthetic data engine promise to reshape data-driven healthcare, keeping privacy, accuracy, and accessibility as its core principles.
To conclude
Through insights shared by industry experts, we’ve uncovered the diverse applications and benefits of synthetic data across healthcare domains. From addressing data scarcity to enhancing predictive accuracy, synthetic data emerges as a versatile tool for driving meaningful change.
While acknowledging challenges such as data biases and legislative constraints, the consensus remains clear: synthetic data holds immense potential to revolutionize healthcare by safeguarding privacy, ensuring accuracy, and facilitating data-backed decision-making.
With solutions like Syntho offers, bringing together all synthetic data generation approaches on one platform, transforming healthcare through innovation becomes much easier and cost-effective.
You can talk to one of our experts personally. Just book a demo, and we’ll gladly address any questions you have.
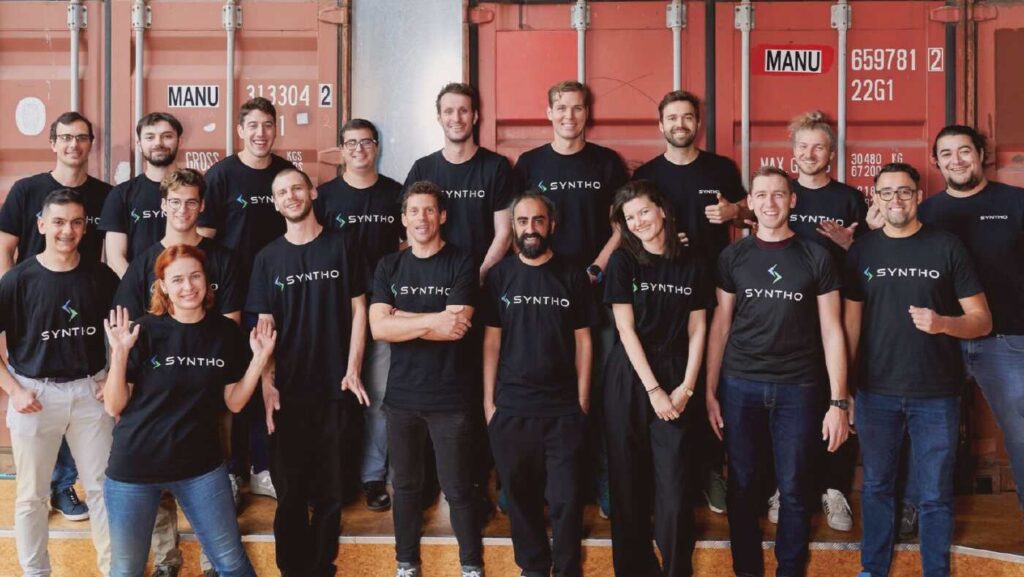
Data is synthetic, but our team is real!
Contact Syntho and one of our experts will get in touch with you at the speed of light to explore the value of synthetic data!